Digital Doppelgängers:
Pseudomorphism as Method
at the Intersection of Technology, Form, and Content
by Leander Gussmann and Alexandra Samoylova
In today's digital terrain, where the distinction between image archives and generative image models blur, pseudomorphism emerges as a beacon. First articulated by Erwin Panofsky1 and subsequently refined by Yve-Alain Bois,2 pseudomorphism offers a means of navigating the complexities of interpreting artworks, conducting archival research, and exploring the potential of generative image-making. We will argue that a balanced approach is needed to better understand visual objects as part of our culture. This approach should combine computational precision with humanistic interpretation. Before exploring how we can use this approach, let's briefly introduce the relevant developments in computer science that provide the necessary technical infrastructure.
Computer Vision
The introduction of Content-Based Image Retrieval (CBIR) systems in computer vision in the 1990s marked a significant shift in visual data categorization, moving from reliance on textual metadata to analyzing images based on their visual content, such as colors, textures, and shapes.3 This shift resonates with formal emphasis in art historical methodologies such as pseudomorphism, which values form over content and examines visual resemblances. Then, the emergence of neural networks, particularly in generative models, fundamentally changed image processing. AlexNet,4 introduced in 2012 by Alex Krizhevsky and colleagues, significantly improved image classification accuracy, revolutionizing computer vision. This development was crucial in using neural networks for visual data interpretation, paving the way for sophisticated image analysis and generation techniques.
Generative Adversarial Networks (GANs), introduced by Ian Goodfellow in 2014, and Neural Style Transfer (NST), developed by Ian Gatys in 2015, represent significant advancements in this field. GANs5 uses two neural networks—a generator and a discriminator—to create realistic images, and NST6 employs deep neural networks to blend the content of one image with the style of another, using convolutional neural networks to measure and minimize the distance between feature vectors of style and content of images.
These technical developments present new research methods for art history. CBIR's ability to process vast volumes of visual data aligns with art historical methods focusing on form and visual differences. At the same time, generative models like GANs and NST provide novel tools for reimagining historical artworks and exploring new interpretations of content and form. Integrating these technologies into art historical methodology enriches traditional practices, offering a reconfigured approach to categorizing, interpreting, and engaging with visual culture.
Defining Concept and Context in Pseudomorphism
Pseudomorphism occurs when artworks share formal similarities without a causal connection. Distinguishing these similarities from those used in art historical comparisons is crucial. As an observation of visual resemblance, pseudomorphism is a tertium comparationis7 of two or more objects resulting from comparative analysis without an underlying content8 match between the compared objects. Therefore, pseudomorphism can be a methodological tool for digital and archival research and related artistic strategies.9 As the compound noun suggests, with its second stem Μορφ meaning form, the primary aspect of comparison is intended to be the form of the object. Bois also pointed this out by defining the context in which Panofsky introduced pseudomorphism as "his most formalist book".10
Art History’s Methodological Dilemma on Form and Content
As we delve into the theoretical and practical realms of art history and visual culture, we traditionally confront the problem of deciding upon the methodological approach to form. Which should we choose: formalism, structuralism, style, or a combination? Is it possible to avoid involvement with meaning and interpretation and to heed Susan Sontag’s statement that "What is needed, first, is more attention to form in art"?11
In his book General Theory of Visual Culture, Whitney Davis illustrates the fluidity of boundaries between the levels of perception of visual objects, even in terms of their formal aspects, without mentioning the content level, which transmutes within each recipient, a phenomenon he terms ‘interdetermination’.12 Traditional approaches based on retrospective comparative analysis, such as formalism, stylistic analysis, iconography, and iconology—all grounded in the principle of tertium comparationis—seem limited in their capacity for observation, often transforming one method into another in the process, which, at some point, makes it difficult for us to be sure if we are perceiving at the same level as another person or if, quite generally, we are not missing out on something that remains veiled.
In Christian art, we find a relevant example in the traditional depiction of angels within the expulsion from paradise scene. According to Genesis 3:24, one would anticipate the representation of a cherub wielding a flaming sword. In the Vienna Genesis (image 1), we can observe an illustrative case where the motif of fire dominates conspicuously, manifesting as a blazing wheel. Another variation in iconography, adhering closely to the notion of ‘cherubim et gladius flammeus,’13 is discernible in medieval art, where the sword is either rendered in a vivid red hue, as demonstrated in the following example, or depicted as burning, with flames visible along the perimeter of the sword. (image 2) The subject modification exemplifies the fluidity inherent in the boundaries of interpretation, a concept elucidated by Davis. To illustrate the fluidity of this motif, we can also examine Masaccio’s renowned depiction of the Expulsion (image 3). The angel positioned in the upper left corner of the fresco neither brandishes a flaming sword nor contains any literal representation of the fire motif. Instead, what is presented is the angel’s reddish attire. This prompts the question of whether we ought to perceive the color of the angel’s garments as an iconographical extension of the flame motif or as a purely stylistic choice. This consideration gains particular relevance when contemplating the entirety of the pictorial cycle. If we interpret it from both perspectives, does this dual perception provide a better understanding of the motif?
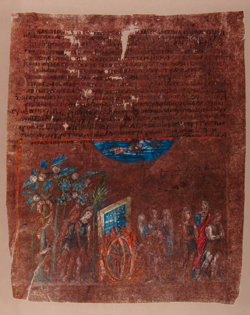

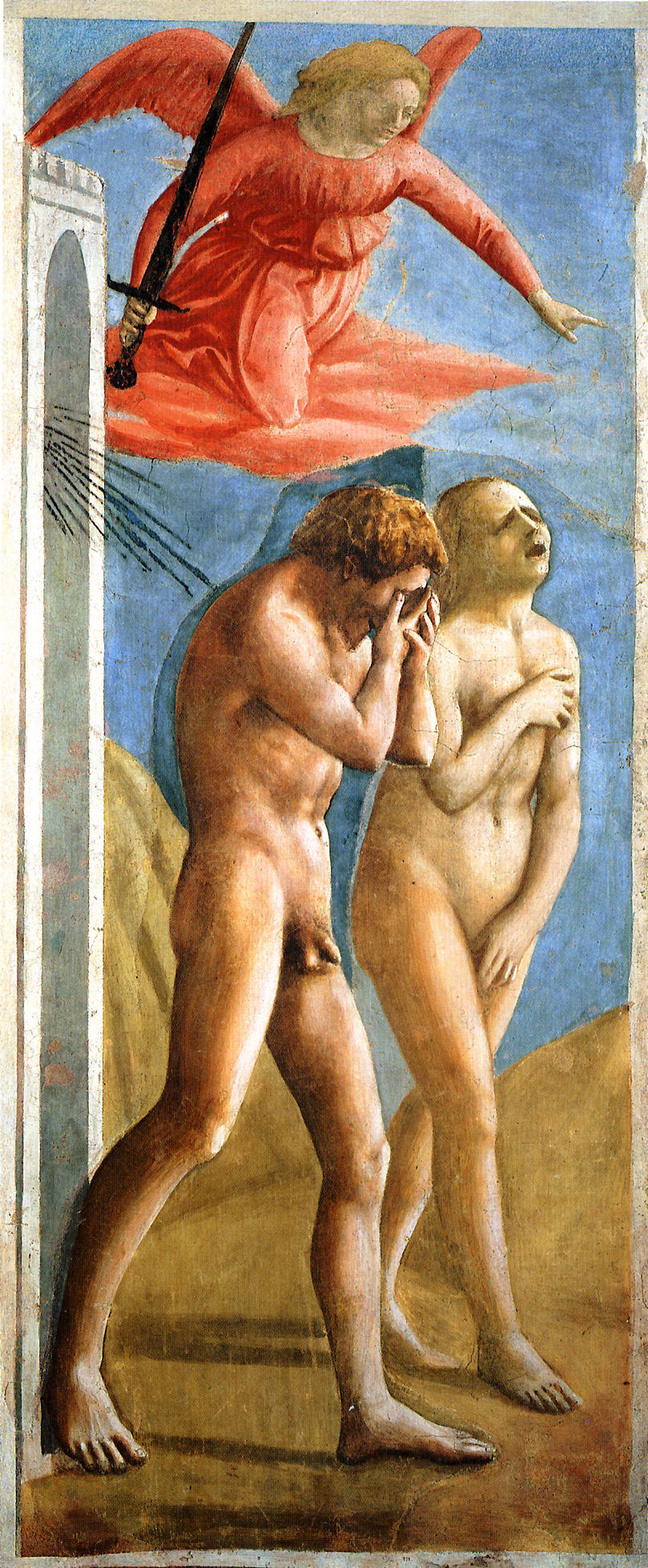
Image 1: Expulsion from
Paradise, Cod. vindob. theol. graec. 31, Fol. I, P. 2, 6 century, Austrian
National Library, Vienna, Austria.
Image 2: Expulsion from
Paradise, MS M. 338, fol. 54r, ca. 1200, Morgan Library and Museum, New York,
USA.
Image 3: Masaccio, Expulsion from Paradise, fresco, 1425–1428, Capella Brancacci, Santa Maria del Carmine, Florence, Italy.
Art Challenges Conventional Form through Formlessness
Expaning upon the definition of form, we can refer to the concept of l’informe, conceptualized and presented by Georges Bataille.14 He discusses not just a lack of form but a force that declassifies, making the familiar strange and disrupting the distinctions that give things definition. Bois and Krauss15 reintroduced the term Formless, in the sense of an aesthetic strategy that artists have adopted, using entropy to engage with the formless through various means, such as materials that degrade over time, practices that involve accumulation or redundancy, or processes that inherently lack precision and predictability. This artistic practice is an oxymoron: any attempt to manifest the formless or make it a subject of study or a representational figure inherently contradicts its nature, raising the question of how to engage with what resists definition without neutralizing its subversive power.
With the critical approaches toward understanding form in the discourse of art history and visual culture outlined, a question arises: To what extent is it possible to adapt pseudomorphism and our definition of form into the practice of digital archival research—and what can art history/visual culture research gain from this adaptation?
We now explore how pseudomorphism could significantly enhance art history and visual culture studies if pseudomorphism were adapted into digital archival research. We test if prioritizing form over content aligns with digital methods, particularly in calculating visual similarity and distance with vectors. Such integration could lead to broader, context-independent comparisons, uncovering new links across artworks and deepening our understanding of visual cultures. Consequently, art history might benefit from a data-driven, objective, and technologically integrated analysis, expanding the research scope and depth.
The Role of Image Retrieval in Digital Art Research
First, we will look at how integrating Content-Based Image Retrieval (CBIR) systems and neural networks, which enable generative models such as GANs, NST, and diffusion models, expands art historical research methods.
Content-Based Image Retrieval (CBIR) is a technology that analyzes an image’s visual content rather than any associated metadata or textual descriptions. However, in practice, most image search systems, including those labeled as CBIR, often use a combination of content-based features and some form of metadata. Using CBIR, we might enhance conventional interpretations, highlighting formal and structural resemblances over the content or historical context. This approach seems opposed to the usual art history practice, which is marked by preliminary contextualization of the object, whether it be a topic of formal manifestation or the form itself. Conversely, by creating a new way of indexing, we risk getting trapped in the same loop of categorical interdetermination that Davis identifies. Davis refers to the philosophy of language, mainly the Wittgensteinian concept of Lebensform,16 which he uses to identify and understand the perspective of someone who observes and interprets an object of visual culture. Contextualizing Wittgenstein,17 Davis discusses various linguistic theories, which focus on the communicational function of language and, therefore, perceptual disjunction, primarily from the recipient’s side.18
When discussing CBIR-driven applications and search engines, one of the communicators is a non-human entity, although created by humans, which complicates the communication process rather than simplifying problem-solving. This issue of computational linguistics, and linguistics in general, should be considered in light of the topic’s overall presentation.
Suppose we pause to consider the functionality of digital research tools for visual objects. In that case, they can precisely analyze vast data and transform visual content creation and interpretation. The computational infrastructure of these models involves manipulating vectors and measuring distances and similarities of vectors in multidimensional spaces. In neural networks and image processing, vectors typically refer to a mathematical representation of an image’s features. Each image is restated into a series of numbers (a vector) in a high-dimensional space, where each number represents a specific feature or characteristic of the image, such as color intensity or edge orientation. The concept of similarity in vectors involves measuring how close or far apart these vectors are in the multidimensional space using distance metrics such as Euclidean, Manhattan, Canberra, or others.19 The closer the vectors are, the more similar the images they represent.
The approach is vital for image classification tasks focusing on recognizing and grouping images based on shared features and for image retrieval systems searching for images by matching feature vectors. George Mather’s study20 examines the changes in European art from the 14th to the 20th century through a statistical analysis of 542 artworks from The JenAesthetics and MART datasets, focusing on the transition to Modern Art between 1878 and 1891. The study employs fractal dimension (FD) and Shannon entropy (SE) as measures. FD assesses image complexity by indicating how much detail is divided into patterns; a higher FD suggests intricate detail. SE measures the unpredictability of an image’s intensity values, with higher values indicating greater complexity and less predictability.
Key findings reveal that FD and SE values were stable,21 akin to natural scenes,22 until the late 19th century, suggesting that art mimicked natural visual statistics. The variability in these measures increased with the advent of Modern Art, indicating a shift in artistic expression. Modern paintings, particularly abstracts, were more likely to deviate from previous art forms, suggesting a change in aesthetic preferences. Beauty ratings correlated with FD and SE before the Modern period but not afterward, hinting at a change in the criteria for appreciating art. The methodology was robust, accounting for image processing factors and strengthening the study’s conclusions.
This study links the rise of Modern Art to a statistical shift in visual complexity and unpredictability, reflecting a broader diversification in artistic expression beyond visual aesthetics. Considering the research subjects, this project is broadly focused on finding mimesis, although the study was more concerned with tracking changes in visual statistics. This additional interpretation, by outlining mimetic aspects, much in the tradition of art history, appears as a further step of interpretation based on the statistical results provided by the study.
This research approach challenges the established methodological approach of art history and visual culture, in which the object of research has mainly been the visual object itself. When the machine assumes the role of mediator between the viewer and the visual unit, the recipient is confronted with the interpretation of statistical data and the adjustment of the results to the existing conceptual framework, which may be a challenging aspect of academic and artistic practice.
Amanda Wasielewski23 points out the general problem of integrating computational methods and data analysis in humanities disciplines, particularly in art history, and the challenges and implications of this integration. She highlights the shift from seeking stable universals to embracing theories and systems which consider specific contexts or frames of reference.
Her key focus is objectivity, often assumed in scientific fields and inherent in computer vision and machine learning. As these techniques are applied in humanities disciplines, Wasielewski suggests that presumed objectivity should be critically examined, especially in digital humanities research. The transformation of culture into data involves translation, interpretation, and representation processes, often underemphasized in quantitative cultural analysis. She argues that data in humanities should be viewed as capta, meaning ’taken,’ in opposition to data, meaning ‘given’,24 to acknowledge its constructed nature rather than being a natural representation of pre-existing facts. This perspective is linked to the earlier stated problem of the communicational chain in the frame of human-computer interaction.
She references media theorist Lev Manovich’s promotion of cultural analytics,25 using computational methods to study culture. Manovich advocates for methods beyond traditional categories, using computational methods to question existing categories, generate new ones, or create new cultural maps. However, Wasielewski counters that data analysis, even with its capability to yield unexpected results, cannot be considered objective due to the biases in data and the challenges in pinpointing these biases in large datasets. This observation extends to formal methodologies like CBIR and pseudomorphism, which, despite appearing objective, are not immune to errors, biases, and distortions.
Computational methods are not fundamentally flawed for studying humanities; they come with ideological baggage. The notion that quantitative research is less subjective and better reflects objective conditions should be challenged. Wasielewski’s analysis emphasizes the need to acknowledge errors, uncertainties, biases, and omissions in data analysis, arguing for a more critical and nuanced approach to integrating computational methods in humanities research.
Indeed, the capabilities of digital tools offer the opportunity to process a vast volume of visual data. Klinke26 addresses the topic of Big Image Data (BID) and the transformative effects of digitalization on art history through it. The article highlights how BID enables the comparison of vast image collections, expanding traditional methodologies like iconography and iconology. As computational tools are integrated, challenges arise due to the complexity of image processing, which is more intricate than text analysis. The nature of images in the digital era necessitates a redefinition, differentiating between the image as visual data and its medium. Big Data’s principles—volume, variety, velocity, and visualization—are crucial for analyzing and interpreting art historical data.
BID’s potential extends art history’s capabilities to predict trends and patterns. However, this necessitates a modernized approach that combines digitized archives with other knowledge sources. Collaboration with information scientists is essential to develop appropriate digital tools. The article advocates for digital literacy in art history curricula, preparing scholars for traditional analysis and digital resource critique. Art historians are transitioning from recipients of images to producers of visual data, for example, by using generative models like GANs and NST to reimagine historical artworks, explore new content, and form interpretations. Such methods are currently not widely used or researched, and they underscore the need to understand, critique, and explore digital image methods. Klinke concludes by emphasizing the importance of digital methods in art history for studying traditional art and contemporary digital-born works, ensuring the discipline’s future relevance.
We talked about reading and interpreting statistical data, and here, we should mention the position of professional recipients regarding the utilization of such images and data-driven technology in their practice. A qualitative research study by Joan E. Beaudoin27 investigated the awareness and potential use of Content-Based Image Retrieval (CBIR) systems among professional image users from various domains, such as archaeology, architecture, art history, and the arts, considering that CBIR systems prioritize the analysis of visual elements such as texture, color, and shape over textual metadata. The study involved twenty participants who relied on images for their work. It found that interest in CBIR varied: professionals who deal with the formal characteristics of images showed the most potential for using CBIR systems, while those in need of images of specific known items, themes, or locations saw text-based searches as more effective.
Regarding artists, the study found that they predominantly favored print and other analog resources, with a marked preference for using images from their personal collections. This preference may influence their skepticism towards CBIR systems, as these systems are digitally oriented and might not align with the artists’ established methods and sources for visual inspiration.
Art historians, in contrast, have been thoroughly researched in terms of their image use. Beaudoin notes that art historians rely on visual materials, often turning to their departmental slide collections, personal collections, museum images, and images they have produced themselves.28 They have also been found to use textbook sets and make their own slides or photographs. Despite this reliance on various image resources, Beaudoin’s study did not find significant interest in CBIR systems among art historians. This might be due to their established research methods and practices, which often involve specific known-item searches where direct, text-based queries are more efficient and practical.
The interpretation of Bedouine’s study brings us back to the statement mentioned at the beginning of the article, namely the preliminary contextualization of a research object in art history. Taking this statement to the edge of absurdity, we must first have a concept of a triangle to identify a triangle. In the context of a digital archive—indexing the data—as Wasielewski mentioned regarding Manovich’s work, we either use existing categories or create new ones. However, we remain in a state of categorical interdetermination in the sense of Davis’s theory. We are confronted with the fluidity of boundaries in the perception process.
Pseudomorphism in the study of modern and contemporary art poses significant challenges. Nevertheless, the adjusted use of pseudomorphism in the research of modern and contemporary art is justified, and it can be used for researching medieval art, which is inductively classified as religious art. Its application may be reasonable and is expected to advance. This development raises a pivotal question within a broader art historical context: Can it effectively apply pseudomorphism and its emphasis on likeness in medieval art history, primarily considered within religious art?
Pseudomorphism and Iconography
One of the ways pseudomorphism might be well adjusted to iconography is by balancing the border between the pre-iconographical and iconographical stages of Panofsky’s method.
Generally, the topic of –morphisms is a recurring theme in medieval Western and religious art’s iconographical research. In art, the term polymorphism, which refers to the various formal manifestations of the same content, like the Holy Spirit appearing as a dove or as flame motifs in the iconographical compositions of Pentecost, or even both simultaneously. The adjusted pseudomorphism, as a tertium comparationis in the sense of iconographical form, where one motif can be connotated and have various meanings, can be seen in one motif in the iconographical composition of the scene of the stigmatization of Saint Francis of Assisi (image 4 and 5). The Christus Seraphicus appears in the sky, depicted with four or six large wings in different open-closed configurations starting from the second half of the 13th century. This form might very easily be found identical to depictions of seraphs or reduced iconographical variants of cherubs as tetramorphs. (image 6) Despite their formal similarity, those motifs are different in meaning.
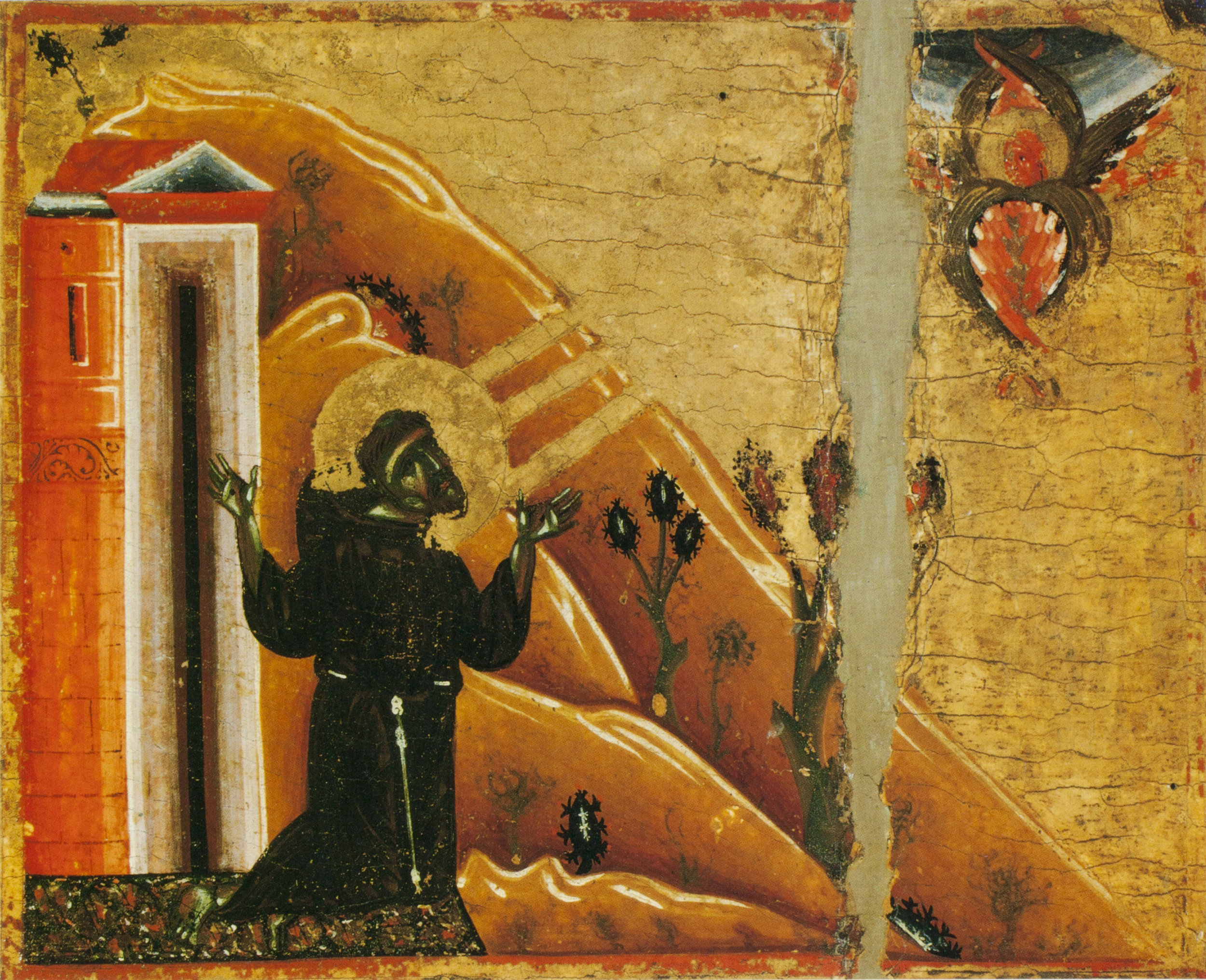
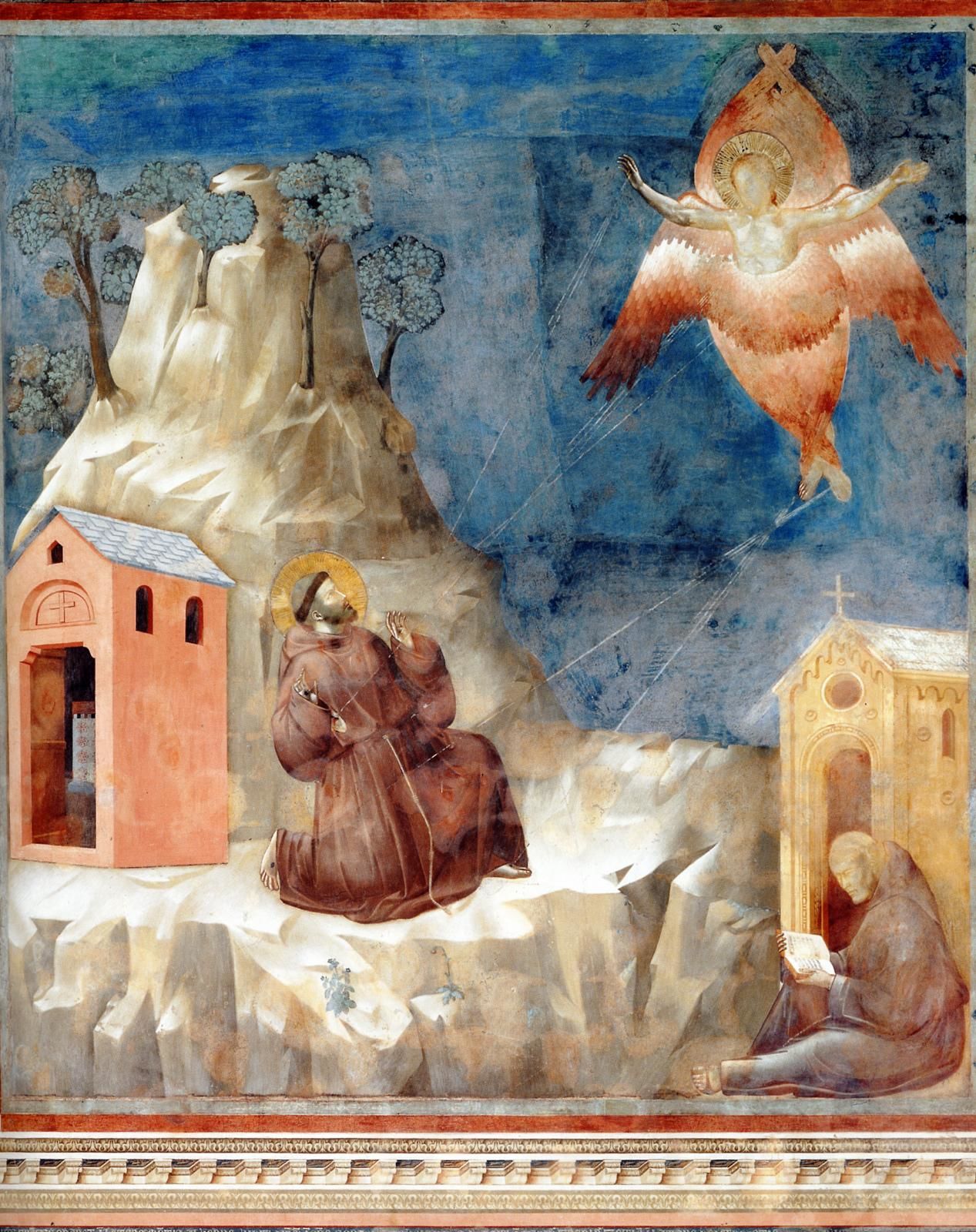

Image 4: Maestro del San Francesco Bardi, Retable Depicting Scenes from the Life of St. Francis, Scene No. 12, Stigmatisation of St. Francis, tempera on wood, 1250 –1260, Capella Bardi, Basilica di Santa Croce, Florence, Italy.
Image 5: Giotto di
Bondone, Stigmatisation of St. Francis, southern wall of nave of the upper
Basilica, Basilica of Saint Francis of Assisi, fresco, 1292–1305, Assisi,
Italy.
Image 6: Seraphs and Herubs, Mosaic on the Cross-Vaulted Ceiling of the Presbyterium, ca. 1145–1160, Cefalù Cathedral, Sicily, Italy.
Pseudomorphism Spanning Medieval to Modern Art
So, regarding the primary condition of defining
pseudomorphism at the beginning as "visual resemblance without underlying
content matching," we might find it adaptable even for iconography-based
methodology if we intentionally separate form from content in our perception.
Analyzing European medieval art, we cannot escape the stringent cultural
construction of Christianity in its rich manifestations, but we can
intentionally filter perception which takes us beyond the form-content
dichotomy. This capacity to filter perception depends on whether we can get
that data analysis from a digital tool or if it remains a further
interpretative task for the researcher.
Descending from the heavenly realm of European
Middle Ages’ visual practices to a more earthly level, we may illustrate
a dichotomous pseudomorphism with the image that Bois discussed in his article:
"Young Woman on the Death Bed" (image 7) by an unknown artist, dated
1612, currently housed at the Musée des Beaux-Arts de Rouen. Bois encountered a
problem categorizing it as an example of Flemish art based on its style and
situated it within the iconographical tradition of depicting the deceased.
Running the image through Google and Yandex picture searches yields not only
generic references to the work itself but also usage of it as an illustration
to various articles and its contemporary iconographical reception through memes
(image 8, especially 9).
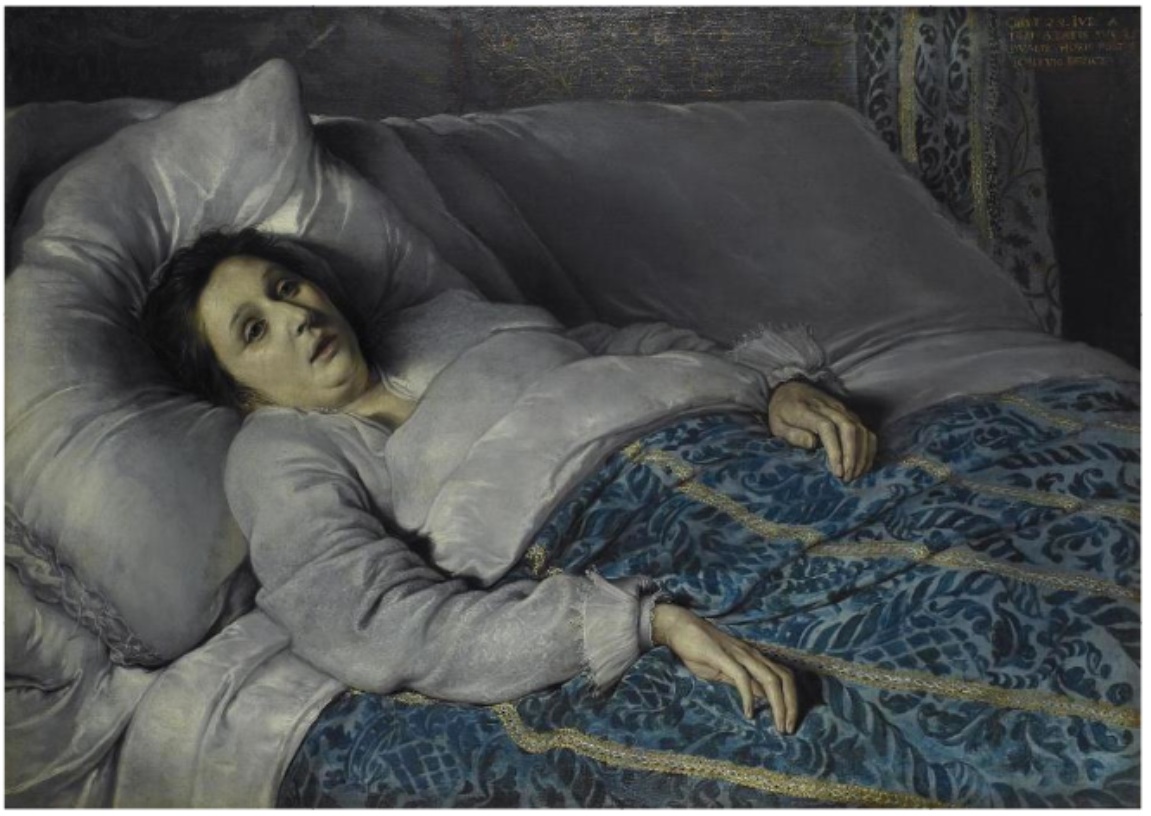
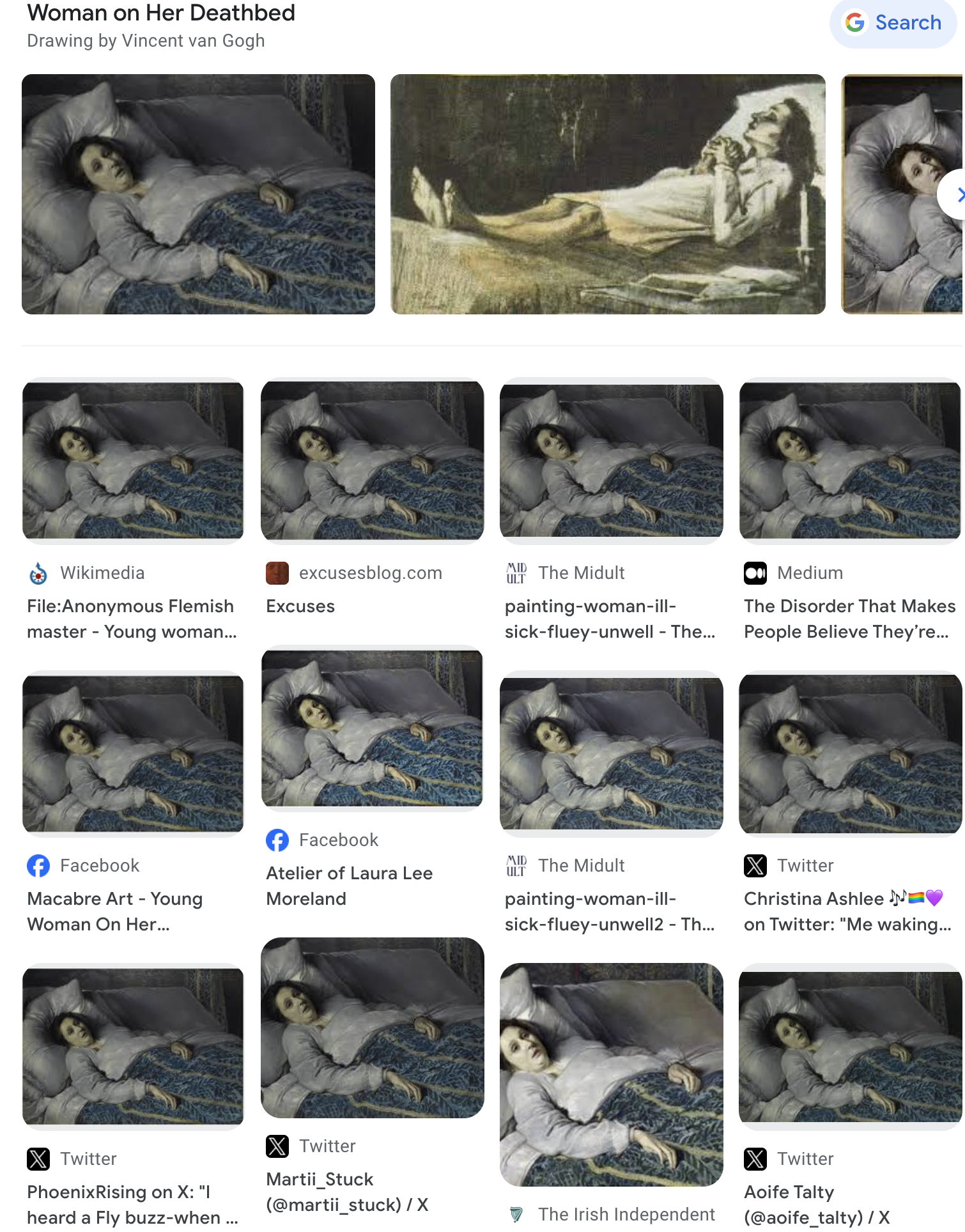
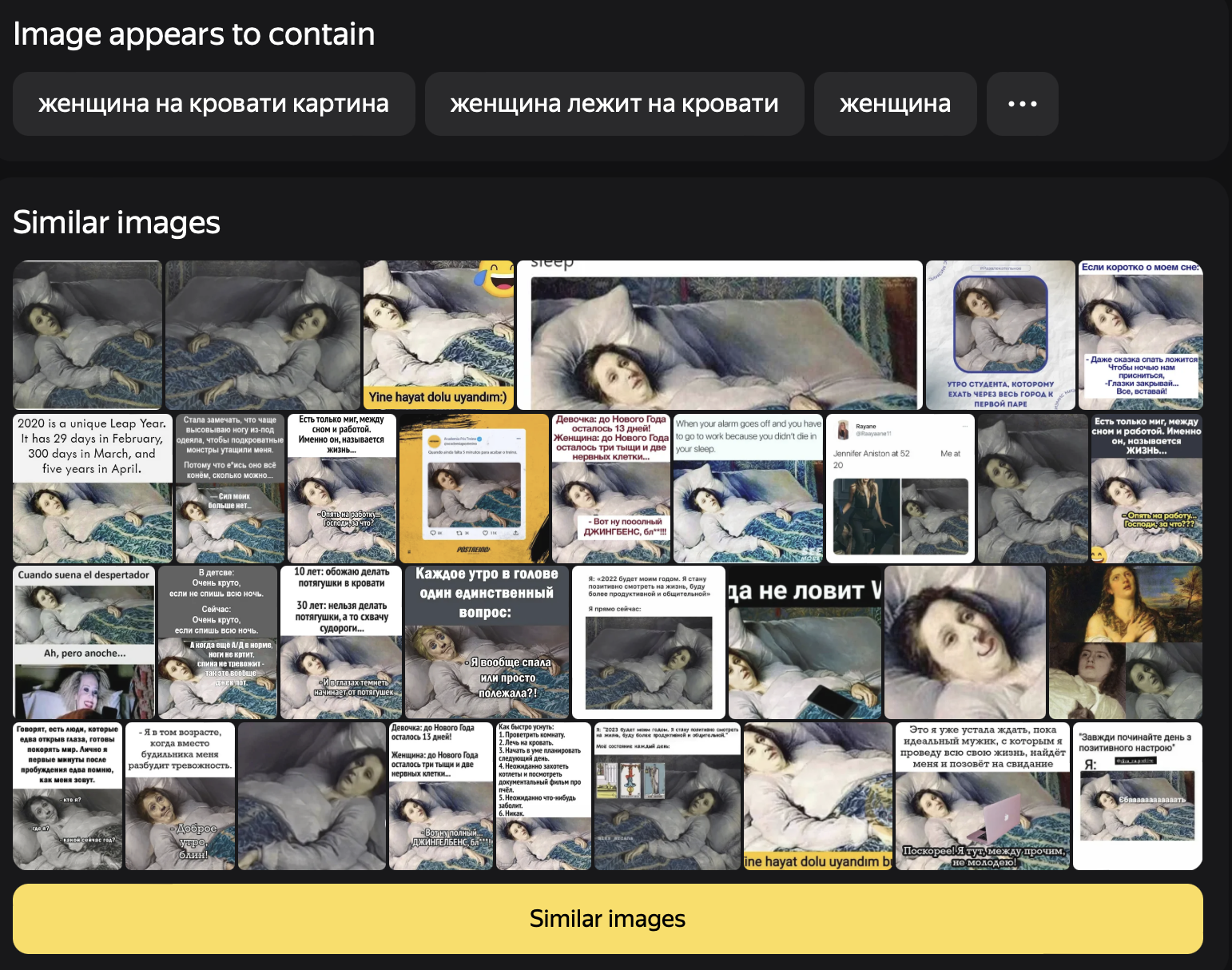
Image 7: Unknown artist,
Young Woman on the Death Bed, 1621, Musée des Beaux-Arts de Rouen.
Image 8: Image search
results with Google lens.
Image 9: Image search results with Yandex
pictures.
Pseudomorphism and the Digital Transformation of Art History Today
In summary, the study of pseudomorphism—through the lens of art history and digital humanities—reveals a complex interplay between form, content, and methodology in interpreting visual culture. It offers a comparative, form-focused analysis distinct from content analysis. While pseudomorphism allows for a comparative analysis emphasizing visual similarities, it deliberately avoids conflating these with content, offering a fresh perspective on archival research and artistic strategies. The advent of digital tools, particularly Content-Based Image Retrieval systems, challenges traditional art historical approaches by shifting the focus toward formal and structural elements. However, this shift also highlights the limitations and biases inherent in computational methods, as highlighted by Wasielewski’s critique of data objectivity and Klinke’s call for digital literacy among art historians. Mather’s statistical study of art transformations and Beaudoine’s research on CBIR systems’ usability further illustrate the potential and pitfalls of applying quantitative analysis to the humanities. As Bataille, Bois, and Krauss discussed, the concept of formlessness within the arts underscores the paradox of engaging with formlessness, pointing to the subversive power of forms that defy traditional categorization. In integrating computational methods into the humanities, we must navigate these complex dynamics, balancing the insights afforded by digital tools with the nuanced understanding that humanistic inquiry provides. The goal is to improve our interpretation of visual objects, not only as isolated artifacts but as interconnected elements within the broader tapestry of visual culture.
Leander Gussmann is a doctoral candidate under the guidance of Diederich Diederichsen at the Academy of Fine Arts in Vienna. In addition to his research, he works as a lecturer in the Department of Expanded Museum Studies and Cross-Disciplinary Strategies at the University of Applied Arts, Vienna.
Alexandra Samoylova has recently completed a Master’s degree in Art History at the University of Vienna. She is focusing on the examination of art through the analytical framework of systems theory, patterns, and algorithms.
1 Erwin Panofsky, Tomb Sculpture: Four Lectures on Its Changing Aspects from Ancient Egypt to Bernini (New York: H.N. Abrams, 1964), 26–27, 52, 54, 62, 82.
2 Yves-Alain Bois, "On the Uses and Abuses of Look-alikes," Magazine Ltd., and the Massachusetts Institute of Technology 154 (2015): 127–149.
3 Smeulders, Arnold W.M., et al., "Content-Based Image Retrieval at the End of the Early Years," IEEE Transactions on Pattern Analysis and Machine Intelligence, vol. 22, no. 12 (2000): 1349–1380.
4 Krizhevsky, Alex, Ilya Sutskever, and Geoffrey E. Hinton, "ImageNet Classification with Deep Convolutional Neural Networks," Communications of the ACM, vol. 60, no. 6 (2017): 84–90.
5 Goodfellow, Ian, et al., "Generative Adversarial Networks," arXiv preprint arXiv:1406.2661 (2014).
6 Gatys, Leon A., Alexander S. Ecker, and Matthias Bethge, "A Neural Algorithm of Artistic Style," arXiv preprint arXiv:1508.06576 (2015).
7 Latin for "the third [element] of comparison", which refers to the shared or common characteristic or criterion that forms the basis for comparing two or more objects, concepts, or phenomena.
8 We use the term ‘content’ in two distinct semantic roles: first, it relates to the 'content and form dichotomy' in art theory; second, it applies to Content-Based Image Retrieval (CBIR) in computer science. It's crucial to understand 'content' within its specific contextual framework, thus precluding any assumptions of its universal applicability across these diverse domains.
9 See Guljajeva, Varvara, Mar Canet Sola, and Isaac Joseph Clarke's (2023) paper, "Artistic Strategies to Guide Neural Networks," in arXiv:2307.07521, for an analysis on the use of neural technology like CBIR, GANs, and NST within artistic practice.
10 Bois, "On the Uses and Abuses of Look-alikes," 127.
11 Susan Sontag, Against Interpretation (New York City: Dell, 1969), 8.
12 Whitney Davis, A General Theory of Visual Culture (Princeton: Princeton University Press: 2018).
13 Latin for “cherubim and a flaming sword”.
14 A concept spread throughout Davis, A General Theory of Visual Culture, for some punctual uses, see: 283, 317–323, 332, 334–335, 340, 352.
15 Yves-Alain Bois and Rosalind E. Krauss, Formless: A User's Guide (New York: Zone Books, 1997).
16 Davis, A General Theory of Visual Culture, 290–292, 317–320, 334–33.
17 Ludwig Wittgenstein, Philosophische Untersuchungen, 19, 23, 241, 11. Edition, (Berlin: Suhrkamp, 2003). Further reading upon the topic, see: Majetschak, Weiberg, Wittgenstein-Handbuch: Leben – Werk – Wirkung, (Berlin, Heidelberg: J.B. Metzler, 2022), 285–292.
18 Davis, A General Theory of Visual Culture, 292–321.
19 Sanjay Patil and Sanjay Talbar, "Content based image retrieval using various distance metrics," International Conference on Data Engineering and Management (Berlin, Heidelberg: Springer, 2010).
20 George Mather, "Visual Image Statistics in the History of Western Art," Art & Perception 6, no. 2–3 (2018).
21 Mather, “Visual Image Statistics in the History of Western Art," 109.
22 Mather refers to the term 'natural scenes' in reference to earlier works by Redies, Hasenstein, Denzler, Ruderman, and Schweinhart. Ruderman was the first to introduce the term 'natural image/scene' in his publication in 1994, which essentially refers to photographs of the real world.
23 Amanda Wasielewski, Computational Formalism: Art History and Machine Learning (Cambridge: The MIT Press, 2023), 23–25.
24 Johanna Drucker, "Humanities Approaches to Graphical Display", Digital Humanities Quarterly, vol. 5, no. 1 (2011): 2.
25 Wasielewski, Computational Formalism, 23–24.
26 Harald Klinke, "Big Image Data within the Big Picture of Art History,” International Journal for Digital Art History, issue 2 (October 2016).
27 Joan E. Beaudoin, "Content-Based Image Retrieval Methods and Professional Image Users," Journal of the Association for Information Science and Technology 67, no. 2 (2016): 350–365.
28 Beaudoin, "Content-Based Image Retrieval Methods,” 358–359.
Illustrations:
Image 1: Expulsion from Paradise, Cod. vindob. theol. graec. 31, Fol. I, P. 2, 6 century, Austrian National Library, Vienna, Austria. P. Buberl, Das Problem der Wiener Genesis, Jahrbuch der Kunsthistorischen Sammlungen in Wien: N.F. 10, Sonderheft 92, 1936, 9–58. H. Gerstinger, Die Wiener Genesis. Farbenlichtdruckfaksimile der griechischen Bilderbibel aus dem 6. Jh. n. Chr., Wien 1931.
Image 2: Expulsion from Paradise, MS M. 338, fol. 54r, ca. 1200, Morgan Library and Museum, New York, USA. http://ica.themorgan.org/manuscript/page/12/158216.
Image 3: Masaccio, Expulsion from Paradise, fresco, 1425–1428, Capella Brancacci, Santa Maria del Carmine, Florence, Italy. Baldini, U.: Die Brancacci-Kapelle, Mailand 1990, 28.
Image 4: Maestro del San Francesco Bardi, Retable Depicting Scenes from the Life of St. Francis, Scene No. 12, Stigmatisation of St. Francis, tempera on wood, 1250 –1260, Capella Bardi, Basilica di Santa Croce, Florence, Italy. Chiara Frugoni, Francesco e l'invenzione delle stimmate. Una storia per parole e immagini fino a Bonaventura e Giotto, Turin 1993.
Image 5: Giotto di Bondone, Stigmatisation of St. Francis, southern wall of nave of the upper Basilica, Basilica of Saint Francis of Assisi, fresco, 1292–1305, Assisi, Italy. La Basilica di San Francesco ad Assisi, ed. Giorgio Bonsanti, Modena 2002 (Mirabilia Italiae 11), 870, Abb. 1709.
Image 6: Seraphs and Herubs, Mosaic on the Cross-Vaulted Ceiling of the Presbyterium, ca. 1145–1160, Cefalù Cathedral, Sicily, Italy. Poeschke, Joachim: Mosaiken in Italien. 300–1300. Hirmer München, 2009.
Image 7: Unknown artist,Young Woman on the Death Bed, 1621, Musée des Beaux-Arts de Rouen.
Image 8: Image search results with Google lens. Retrieval date: 2023-11-10
Image 9: Image search results with Yandex pictures. Retrieval date: 2023-11-10